OP08 Automatic detection of enteric ulcers and erosions in capsule endoscopy using a convolutional neural network
SaraivaJr., M.(1);Afonso, J.(1);Ribeiro, T.(1);Cardoso, H.(1);Ferreira, J.(2);Andrade, P.(1);Jorge, R.(2);Lopes, S.(1);Macedo, G.(1)
(1)Centro Hospitalar Universitário de S. João- EPE, Gastroenterology, Porto, Portugal;(2)Faculdade de Engenharia da Universidade do Port, Mechanical Engineering, Porto, Portugal
Background
Capsule endoscopy (CE) is recommended for the diagnosis and assessment of disease extension in patients with suspected or known inflammatory bowel disease, particularly Crohn’s disease. Ulcers and erosions of the enteric mucosa are prevalent findings in these patients. They frequently occur together, and their identification in CE is crucial for an accurate evaluation of disease severity. Nevertheless, reviewing CE images is a time-consuming task, and the risk of overlooking lesions is significant.
Over the last decade, artificial intelligence (AI) has emerged as a mean for overcoming these pitfalls. Of all AI methods, convolutional neural networks (CNN), due to their complex multilayer architecture present the best results in image analysis.
We aimed to develop a CNN for the automatic identification of ulcers and erosions in the small bowel mucosa.
Methods
A total of 1483 CE exams (PillCam SB3®) performed at a single centre between 2015-2020 were analyzed. From these exams, a sum of 11588 frames of the enteric mucosa were obtained, 3163 containing enteric ulcers and erosions, and the remaining containing normal mucosa or other findings. Ulcers and erosions were stratified according to the Saurin’s classification for bleeding potential: P1E – erosions with intermediate bleeding risk; P1U – ulcers with intermediate bleeding risk; P2U – ulcers with high bleeding risk. For automatic identification of these lesions, these images were inserted into an CNN model with transfer learning. Subsequently, the performance of the network assessed using an independent set of images. The output provided by the CNN was compared to the classification provided by a consensus of specialists (Figure 1).
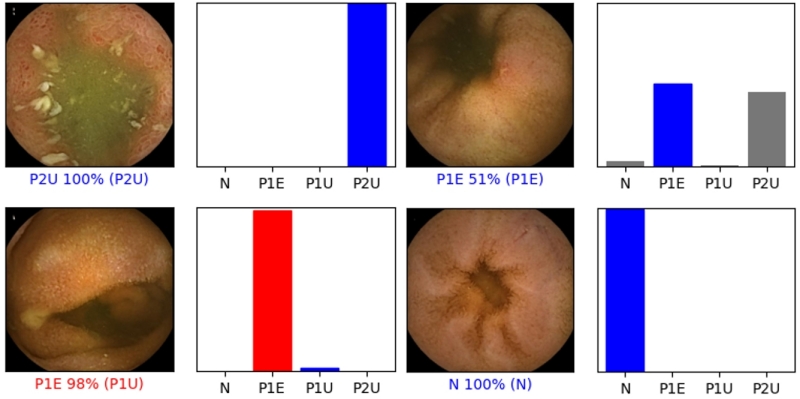
Results
After optimizing the neural architecture of the algorithm, our model was able to automatically detect and distinguish ulcers and erosions (any bleeding potential) in the small intestine mucosa with an accuracy of 96.7%, precision of 95.9%, sensitivity of 91.7% and a specificity of 97.8% (Figure1). The mean processing time for the validation dataset was 23 seconds (approximately 101 frames/second).
Conclusion
We developed and tested a deep learning model based on a CNN for the automatic detection of enteric ulcers and erosions in CE images. Our system revealed extraordinary performance marks. We believe that our study lays the foundation for the development and application of effective AI tools to clinical practice. These techniques should improve diagnostic accuracy and reading efficiency.