P137 Preliminary validation of a multi-stage machine learning algorithm to assess histological inflammation in inflammatory bowel disease
Hagendorn, E.(1);Karsen, S.(2);Pai, R.(3);Jairath, V.(4);Knight, H.(2);Schwartz, A.(2);Laroux, S.(2);Butler, J.(5);Dunstan, R.(2);
(1)AbbVie Inc., Bioinformatics, Worcester, United States;(2)AbbVie Inc., Immunology, Worcester, United States;(3)Mayo Clinic Arizona, Department of Laboratory Medicine and Pathology, Scottsdale, United States;(4)Western University, Department of Medicine Division of Gastroenterology, London, Canada;(5)Former AbbVie Inc. Employee, Immunology, North Chicago, United States
Background
The histologic assessment of inflammatory bowel disease (IBD) relies on qualitative grading methods. Although widely accepted, these instruments are time consuming, require specialized training, and suffer from inter-rater disagreement. For these reasons there is a need for more consistent and less biased methods to assess IBD histology.
Methods
The algorithm was initially developed using hematoxylin and eosin (H&E) stained whole slide images of colon biopsies (238 ulcerative colitis [UC], 30 Crohn’s Disease [CD], and 28 endoscopically normal adjacent [ENA]). The first two stages implement convolutional neural networks (CNN) which segment 11 key anatomical features (Figure 1). The third stage extracts the features and models them for prediction.
Figure 1: Segmentation results for stage 1 at 5X (left) and stage 2 at 20X (right).
Results
The first stage of the algorithm was validated on an independent test dataset by calculating the intersection-over-union (IoU) for the ground truth and prediction masks, resulting in a value of 0.97. A preliminary validation for stage 2 was performed by randomly selecting 30 unique biopsy sections from the test dataset and applying a 150um x 150um counting frame. An expert gastrointestinal pathologist confirmed correct cell identification by the algorithm for three of the primary inflammatory cell types: plasma cells, eosinophils, and neutrophils which resulted in a sensitivity/specificity of 0.76/0.99, 0.78/1.00, and 1.00/0.98 respectively. The final stage predicts RHI grades which could be directly compared to pathologist reads (Figure 2, 3, and 4).
RHI Score | Description | Clinical Importance (Total=1.0) | Sensitivity | Specificity |
---|---|---|---|---|
Grade 1 | Chronic inflammatory infiltrate | 0.09 | 0.99 | 0.50 |
Grade 2B | Neutrophils in lamina propria | 0.18 | 0.87 | 0.92 |
Grade 3 | Neutrophils in epithelium | 0.27 | 0.97 | 0.88 |
Grade 5 | Erosion or ulceration | 0.45 | 0.82 | 0.92 |
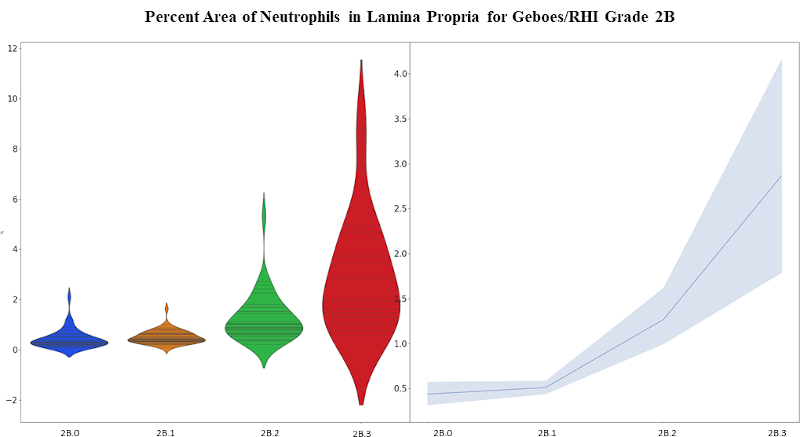
Figure 3: Violin plot (left) and mean with standard error plot (right) showing the increase of neutrophils within the lamina propria for Grade 2B (neutrophils in the lamina propria).
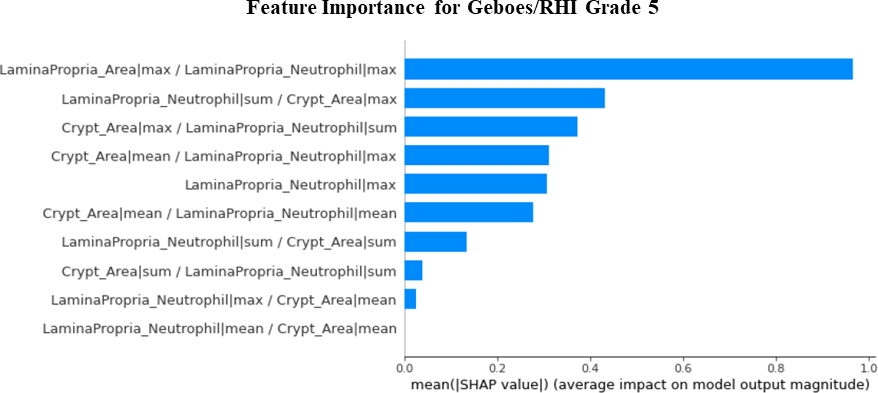
Figure 4: Machine learning model explanation for Grade 5 (erosion and ulceration) predictions revealing a strong reliance on neutrophils in the lamina propria.
Conclusion
This is the first study to demonstrate the value of machine learning to assess histologic activity in IBD. These methods lay the foundations for future work, and we believe stages 1 and 2 can be explored independently to statistically characterize the histologic changes of IBD, enabling the improvement of preexisting grading systems.